The U.S suicide rate is at a 30-year high. According to the National Center for Health Statistics, in 2014 (the last year for which figures are available), 42,773 Americans took their own lives, most of them men.
It’s a crisis, one mental health professionals have historically been ill-equipped to handle. Last year, Joseph Franklin (then a postdoctoral fellow at Harvard, now an assistant professor of clinical psychology at Florida State University) looked at 365 studies on suicide over the past 50 years and found that someone flipping a coin had the same chance of correctly predicting whether a patient would die by suicide as an experienced psychiatrist — 50/50.
If humans are so mediocre when it comes to gauging suicidal intentions, could machines be better? Signs point to yes. IBM’s Watson supercomputer diagnosed a rare cancer doctors missed, while in England, the National Health Service is trying out Google’s DeepMind artificial intelligence for everything from diagnosing eye illnesses to finding out how best to target radiotherapy.
The link between A.I and mental health is less hyped, but Franklin and his team have developed algorithms that can predict whether someone will die by suicide with over 80 percent accuracy. He hopes they may soon become standard, in the form of software that every clinician has access to — and thus help save lives.
What made you want to study suicide prediction?
When I got into suicide research, I wanted to look at everything and see where we were. My hope was that would provide me and my colleagues with some more specific direction on what we knew and could build on. And what we found was quite surprising. We figured out that people have been doing this research where we’ve been very bad at predicting suicidal thoughts and behaviors and we really haven’t improved across 50 years.
Are there common misconceptions about suicide risk?
A lot of people believe that only someone who is showing clear signs of depression is likely to have this happen. I’m not saying depression has nothing to do with it, but it’s not synonymous with that. We can conservatively say 96 percent of people who’ve had severe depression aren’t going to die by suicide.
Most of our theories which say this one thing causes suicide or this combination of three or four things causes suicide — it looks like none of those are going to be adequate. They may all be partially correct but maybe only account for 5 percent of what happens. Our theories have to take into account the fact that hundreds if not thousands of things contribute to suicidal thoughts and behaviors.
More men take their lives than women, but more women attempt suicide. Are there any theories why?
One thing people point to now is something called suicide capability, which is basically a fearlessness about death and an ability to enact death, and one assumption is that men, particularly older men, may be more capable of engaging with these behaviors. But evidence on that right now is not conclusive.
Are traditional risk assessments getting some things right?
Talking to people, not making it this taboo subject, I think that’s great. The problem is we haven’t given them much to go on. Our implicit goal has often been to do research so we can tell clinicians what the most important factors are, and what we’re finding is that we’re just not very accurate.
What we’re going to have to do is this artificial intelligence approach so that all clinicians are able to have something that automatically delivers a very accurate score of where this person is in terms of risk. I think we should be trying to develop that instead of, you know, “these are the five questions to ask.”
How does artificial intelligence predict who is most at risk?
We took thousands of people in this medical database and pored through their records, labeled the ones who had clearly attempted suicide on a particular date and ones that could not to be determined to have attempted suicide, and we then let a machine-learning program run its course. We then applied it to a new set of data to make sure that it worked. The machine has now learned, at least within this particular database of millions of people, what the optimal algorithm seems to be for separating people who are and are not going to attempt suicide.
What kind of data is being fed into the algorithms?
They look at demographic factors: how old are they, what gender are they, there’s something called the zip code deprivation index where you can see is this a wealthier area or is this an economically distressed area, also things like medication history. Men over 45 who have been hospitalized for psychiatric issues, own a gun and are recently divorced are at higher risk than young women who have great jobs and have never had a psychiatric problem. But within these general categories, there are hundreds or even thousands of factors that may contribute to risk, although all contribute inconsistently and in a fairly weak way.
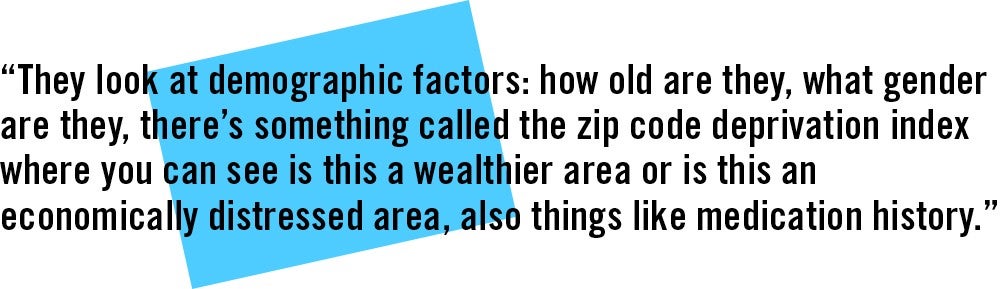
Something we’re working on right now is natural language processing, where you can have the algorithm go through all the notes in health records and pick out certain terms or constellations of terms. That can be a richer set of data: a code for depression but also descriptions of what might be going on for them at that point in time. What the algorithm then does is take all this specific information and combine it in a very complex way. It can take very odd, complicated combinations of hundreds of factors that are just beyond how most of us would think.
And how accurate is it?
It depends. If we’re just taking anyone who walks into the hospital it’s somewhere around 91–92 percent accurate. And if we’re taking people who have some kind of self-injury, so things like accidental drug overdoses or non-suicidal self-cutting, then it’s somewhere around 86 percent. Basically, the more similar two groups are, the harder it is to pick them apart. The greater the number of differences between two groups, the easier it is for an algorithm to sort them into those groups.
Could it get to 100 percent?
I think certainly it can get to 98–99 percent. Getting fully to 100 becomes a question about whether we have free will.
How long will it be before doctors can use artificial intelligence with their patients?
In other areas of medical research, they’ve found that no matter how good the algorithm might be for saying, “This patient definitely needs this drug” or “you may need to look out for this,” it’s hard for a doctor to know whether to trust that. So there’s a lot of questions around how to best present the information in as usable a way as possible to clinicians, and hopefully in a year or two we’ll have some answers.
Once people at risk of suicide have been identified, how can they best be helped?
There’s evidence showing that when gun regulations are passed in specific areas the suicide rate tends to go down, but in terms of psychological interventions, there’s much less robust evidence. We know that a few different types of techniques may work somewhat for some people but there’s nothing that’s close to being a panacea.
What our group has also done is try to develop app-based treatments for suicide and self-injury and we’ve had some success with a particular app, Tec-Tec. People who engage in non-suicidal self-injury and suicidal self-injury tend to not mind images and words related to suicide, death and injury, so what we wanted to do was to condition them to find that more negative. We did that through something called evaluative conditioning, which is just pairing certain types of images and words — in this case very unpleasant ones — with pictures of death, suicide and injury, and over time that changes how people feel about that concept in general.
On the other end, we wanted to improve people’s associations with the self by changing their interpretations of words like “me,” “myself” and “I,” which we found people who engage in self-injury evaluate very negatively, so this matching game conditions more positive associations with that. Between self-cutting and suicidal behaviors, it will drop those between a third and a half over the course of a month. This was actually the first intervention shown to reduce non-suicidal self-cutting and suicide plans, but there’s more research to be done before I would recommend that as the answer.
It sounds like you’re hopeful that you’ll find some solutions.
That’s definitely a message we’re trying to send—that help is on the way. We didn’t make a lot of progress on our big goals in the psych field over the last 50 years, but in the last two or three, we’ve had some huge shifts. We’re all hopeful that within the next few years we’ll be able to start implementing those to have real effects for people in need.
Within the next decade, I envision a proliferation of effective app-based treatments, or whatever the technology is in 10 years, identifying people on a large scale very actively, possibly through social media data, and then immediately connecting them with whatever kind of intervention might be most appropriate online in a quick, easy, free format. I think if we can ever get to that point, of having scalable and accurate risk detection and scalable and effective risk prevention, then I think that’s where we’ll start to see huge changes.
The National Suicide Prevention Lifeline provides free, confidential emotional support 24 hours a day. Call 1–800–273–8255, or see Suicide.org for help outside the U.S.